“Information is the oil of the 21st century, and analytics is the combustion engine.” (Peter Sondergaard, Gartner Inc.).
Data Analytics is a kind of approach that turn data into insights. Let us consider a reputed sport shoe brand that sold its products through an online shop. The manufacturer of that sport shoes runs its business through digitalized solution, and every customer order is tracked in a database, including the type of the shoe, the name of the customer, the price, and so on. With Data Analytics, we can use the data stored in the database to generate insights. For example, we could easily categorize customers and match the customer’s shipping address with a map to check in which location most of the shoes are sold, or we could calculate key performance indicators (KPIs), such as the average delivery time, the costs per order, or the ratio of orders that are processed automatically (without manual touches in the process.
This is the value of Data Analytics, as stated in the opening quote: information is the oil and analytics uses that information to produce a valuable result, like a combustion engine that converts oil into energy.
The Challenge
Although there is an obvious value of Data Analytics, this value is often restricted. Business Intelligence (BI) is one of the ways to implement Data Analytics. BI focuses on creating reports, calculating KPIs, and supporting decision making through dashboards. Another way is, Process Mining that uses event log management of IT systems to visualize the business processes run and sort out the inefficiencies.
See Figure 1 for an example.
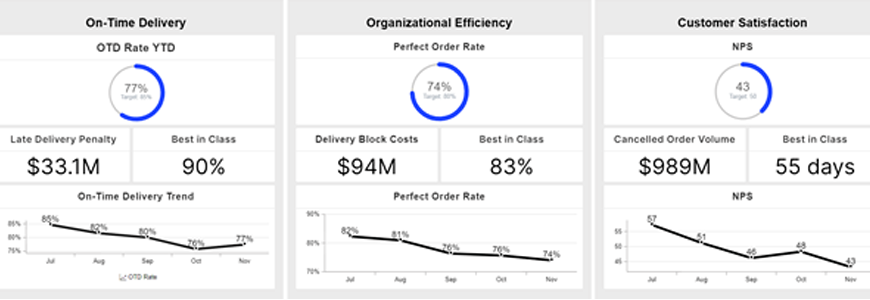
Figure 1. A dashboard that shows specific KPIs in order management, such as the number of cases when a company delivers goods to its customers on time (On-Time-Delivery Rate), the number of orders that have not to be changed manually (Perfect Order Rate), or the customer satisfaction measured by the Net Promoter Score (NPS).
Data Analytics is like an X-ray for a doctor. It shows the strengths and weakness of a business. But what is often missing is, a therapy to solve the problem diagnosed by the X-ray. The X-ray itself would be worthless without a doctor who interprets the results in a right way and uses his knowledge and experience to recommend the best therapy. For Data Analytics this requires going beyond KPIs, reports, dashboards, etc., but also providing an answer to what is next and how to use insights to actually improve.
Consider the sports shoe manufacturer, knowing that 77% of the customers did not receive their shoes on or before the promised delivery date.
We must look into the actions on how we need to take to make sure that we always deliver the shoes within the promised delivery date.
Driving Action
The next step that makes Data Analytics truly powerful is deriving action from the results. That’s when we move it from analytics to real operational support. Many new technologies in the field of automation, machine learning, or artificial intelligence provide promising avenues to take action based on Data Analytics insights.
- Root Cause Analysis: It is not enough to know the status quo. What counts to drive action through Data Analytics is to know “Why”. For the shoe manufacturer, it is good to know that 23% of the customers do not receive their shoes on time. But more importantly, why are they not receiving their shoes on time, what is causing this problem? Are customers in remote areas are specifically affected where the delivery service cannot reach them within the promised delivery date, is the delivery time longer than expected at certain times of the year (e.g., during the Christmas season), or do we have some inefficiencies in our warehouse? Exploring these root causes is the key to derive the right actions for improvement.
- Automation: The insights from Data Analytics could be used to trigger automation. In the case of the shoe manufacturer, the customers who did not receive their orders within the promised delivery date should be found; it should have an automated delivery block in the system if they exceed a certain monthly sales limit and this delivery block has to be checked and released manually by an order manager. But imagine you have very loyal customers who order multiple times a month and always pay on time. For the loyal customers, the order manager checks their order and releases their order block? With automation technologies like Robotic Process Automation (RPA) or general backend automation, we could set up a scenario that would be triggered every time when a customer order is blocked. If that block is for a high-value customer who always pays their invoice on time, we could use automation to release the delivery block automatically and only scan and deep check orders of customers who have not paid their invoice on time.
- Prediction and Simulation: The insights from Data Analytics can be used as input for predictions and simulations. For example, we could use machine learning to predict the expected sales numbers of shoes in the upcoming month, all the information would be taken into account about past sales.
Also, we can even simulate different sales scenarios, for example, the investments we make in advertising or the weather forecast that could affect sales on certain types of shoes. Prediction and simulation then allow us to make the best decision and take action for our business.
In summary, Data Analytics is just the first step. It shows you what’s there. But to create sustainable value through Data Analytics, it needs to be actionable. So, the next time you analyze data, consider how you can take action and make a real impact through Data Analytics for your business.
About the Author
Jerome Geyer-Klingeberg is Head of the Celonis Academic Alliance and responsible for the global research and education program at Celonis. He is also an educator at several universities and published 30+ journal articles, conference papers, and book chapters in the field of Process Mining and other fields of Applied Data Analysis.